© Copyright 2025 — All rights reserved — Art of Visualization
Data Visualization Best Practices to Use in Tableau
Monday 8, November 2021
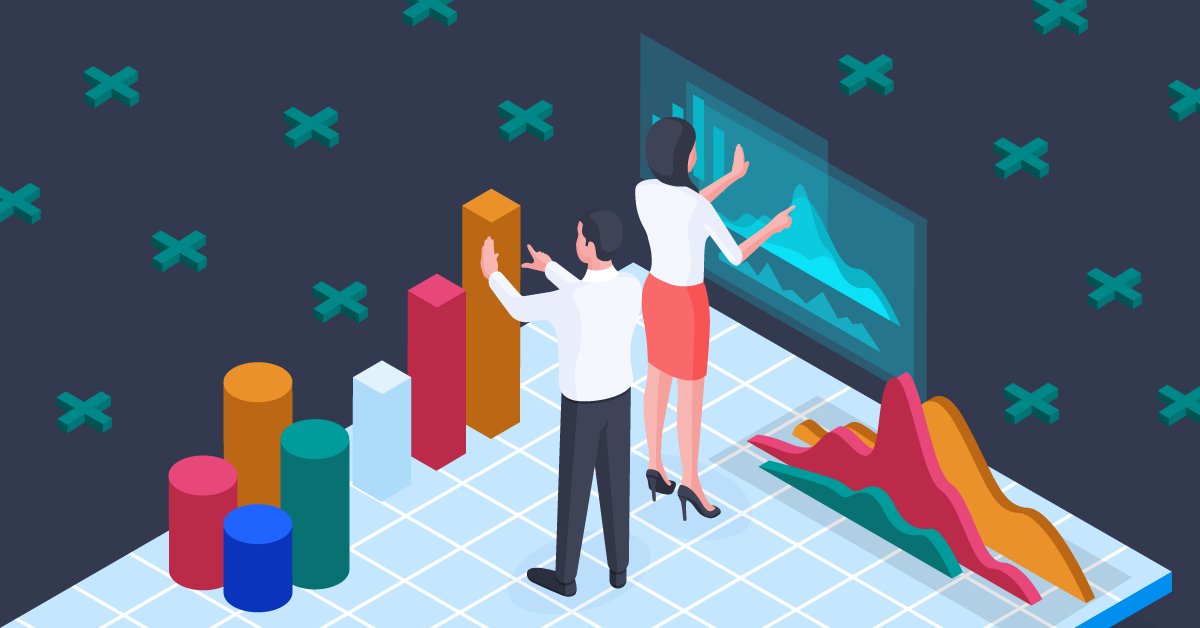
The significance of data today is immeasurable. Data has helped in building new communication tools, metadata layers, and insight automation for Business Intelligence. However, each innovation would have been impossible without Data Visualization best practices.
In this blog post, we take you through the best practices professional data scientists follow to create impressive Data Visualizations. So, steal these golden tips and tricks to take your data storytelling to the next level.
But before you start swimming in the visualization sea, why not take a pool dive to learn...
What is Data Visualization?
Data Visualization is simply the representation of data using visual elements such as graphs, charts, and maps. The purpose is to effectively see, understand, and interpret data trends, outliers, and patterns.
In Business Intelligence, there are several ways to plot and visualize data — Tableau is one of them. So, effective Data Visualization in Tableau is the plotting of data to achieve a comprehensive visual effect using Tableau as a visualization tool.
We need data to analyze, predict, and improve behaviors, trends, and patterns. Data, therefore, is an essential part of life and living. We heavily rely on data to inform everyday decisions, ensure innovation, and lead the way to inventions.
But when data becomes huge, it gets complex to use. As the number of data increases, more people are willing to use the different trends in Data Visualization they could find, such as Tableau, to design insights and deploy them for business growth and development purposes.
What are the types of Data Visualization?
There are two basic types of Data Visualization based on the structure of visualization and not the methods. These two types are static and interactive visualization.
Static Data Visualization
Static Data Visualization suggests the presentation and visualization of data through only one viewpoint or perspective. This form of visualization implies how data can only be seen from a keyhole or a single standpoint.
Static generally means unmoving, so this type of Data Visualization is focused on providing a single story or narrative. Effective Data Visualization examples for static visualization are infographics. Consumers of infographics cannot explore the visualized details beyond what's presented to them.
Interactive Data Visualization
Interactive Data Visualization is not restricted to only one viewpoint; instead, it's focused on multiple viewpoints. As the name implies, users can explore multiple aspects of a story and even customize them to suit their narratives.
Most Interactive Data Visualization concepts work as web applications and make use of interactive dashboards to improve the way we interact with information. Interactive visualization is more fun than static visualization as it provides more options for visualizing information.
Why is Data Visualization important?
Data visualization is important in many ways as it provides a clear direction of what data looks like by providing visual context. Data can be boring, but visual data cannot. Data in its raw form is like pieces of bones. But when raw data are visualized, they become fleshy and adorned in clothes. Below are other reasons Data Visualization is important:
Easy to understand
Data
Visualization not just provides visual contexts but also allows us to quickly understand the nature of data. Through the graphic representation of data, we know what a given data says by engaging with its most intrinsic features and exploring its endless possibilities.
Managers and decision-makers determine sales and metrics using trends in Data Visualization. Indeed, it's easy to read metrics and highlight anomalies when data is presented in visual forms. Also, business managers can find out operating circumstances from multiple perspectives.
Storytelling
Who doesn't love a great story? Clearly, not us. Through Data Visualization, we can visualize data and narrate their stories. Mostly, data is often seen as computational and not narrative. But thanks to Tableau, users of data can turn their dashboards into creative stories.
Tableau turns data into graphics and diagrams to create stories. These stories are then analyzed using Analytics. Conveying data stories is more engaging and interactive than presenting data in primitive forms. Narrated data will always remain indelible in the mind.
Fast and efficient
The human brain functions on visual data faster than textual data. So, plotting data on graphs, charts, and maps is fast and efficient for the human brain to process. It's also great for analyzing data, interpreting it, and implementing.
The swift understanding of data means decisions are made easier and faster. It's one of the Data Visualization rules. Rather than spend hours on corporate meetings deciding what to do with provided data, Data Visualization speeds up the process through graphical presentations and representations.
Patterns and trends
What makes a good Data Visualization is how it spots emerging trends and patterns and responds intelligently to them. It informs analysts about the premise of decisions on rationality when influenced by past decisions (Rational Hypothesis Theory). Likewise, it reveals the Adaptive Market Hypothesis during heightened market volatility.
What are data visualization techniques?
Data Visualization techniques are the visual elements that make it easy to see and understand trends, outliers, and patterns. A good visualization technique should recognize connections within complex data and make it easy for the audience to understand. The five most common Data Visualization techniques include:
Charts
A chart is a graphical representation of data that consists of tables, diagrams, and pictures. Data in charts are implied through symbols such as bars, lines, and slices. A chart can take in tabular numeric data, provide qualitative structure, and reflect the needed details. Common charts include:
Bar Chart
Show the relationship between variables using the height and length of lines or a symbol called bars with rectangles of equal length.
Pie Chart
Shows the relationship between variables using a segmented or sectoral circle. Each segment in the circle represents a calculated portion of the whole.
Line Chart
This shows the relationship between variables using straight lines. Each line tracks current and recurrent changes over time and informs of their performance.
Graphs
A graph is a diagram that shows the relationship between variables, usually two variables. The relationship measures the axes at right angles for each variable. Here are examples of graphs in Data Visualization:
Histogram
Organizes data points into specific variables and ranges. Histograms provide a visual interpretation of discrete or continuous data.
Pictogram
Also known as pictographs, pictograms illustrate the graphical representation of data through pictures. It deploys icons to identify with numbers.
Data Plots
Data plots reflect data values in columns, lines, slices, and other graphical data formats. Plots differ from graphs in the sense that they are used for a finite set of points. The most common plots in Data Science are:
Scatter Plot
Observes relationships between variables by using dots to indicate numerical values through horizontal and vertical axes.
Box and Whisker Plot
Shows the spread of datasets using a boxplot with extended lines to measure the quartiles of groups of numerical data.
Maps
Mapping in Data Visualization analyzes and presents data information in the form of maps. The data is geographically related and contains colors that point towards geographic regions. Examples of map visualizations in Data Science are:
Tree Maps
Visualizes hierarchical data through nested rectangles that indicate corresponding values. Treemaps are space-effective; they contain thousands of items simultaneously.
Heat Map
Shows the magnitude of behaviors as colors. The variation in colors is defined by color intensity to exhibit a significant difference between variables.
Diagrams
Diagrams in Data Visualization are drawings or figures that illustrate the relationship between variables. Diagrams can be simple or complex drawings. They are used to properly dissect data and drive home data narratives. Key diagrams in Data Science are:
Venn Diagram
Shows the relationship between finite variables by using overlapping circles. The overlapped circles tell if variables are similar or different.
Sankey Diagram
Illustrates the flow rate of variables in which the width of a given variable is proportional to the represented value or quantity.
Chord Diagram
Graphically highlights the relationship and inter-relationship between several nodes to show flow rate. It's a type of network diagram.
What are Data Visualization best practices, tricks, and tips?
While the misrepresentation of data could be one of the most unpardonable acts on earth, the poor presentation of data is the same. If you're not presenting data in the most elegant ways, adopting Data Visualization methods and effective Data Visualization principles, you would be hurting your readers.
We've compiled the best 10 tips, tricks, and Data Visualization best practices for you to take advantage of and leverage for data justice.
1. Use the right sources of data
The right sources of gathering data will lead you to the best data stories. There's no story without data, so you have to choose carefully. Whether you're choosing an external source of data or an internal source, you need to be certain your data is clean. Although external data can be engaging, internal data is proven to be more unique and exciting for data storytelling.
2. Choose the chart that tells the story
Your true storytelling power lies in how well you can craft them using the right graphs and charts. There are several visual elements to choose from, so you need to be sure of the one you're using. Some factors you can consider include:
- Your type of data
- Purpose of your story and
- Readers of your story
3. Choose the right tool for your story
Today, you can visualize data in several formats. There are tools with incredible features that help you achieve your data message. However, choosing the right tool is an art. If you're unsure which tool to go for, we recommend Tableau.
4. Design the story for comprehension
Once you've visualized your data, double-check to see if any elements should be added. Always ensure you go for the most simple and effective visual elements. Don't just think about what is to be added, also consider what is to be removed, tweaked, and modified. Each design move you make contributes to the overall design goal you want.
5. Watch your placement
Placements are part of the design. They allow you and your readers to compare and contrast data points. If your bars in charts are too close Your placement should not be too close to or too clustered to avoid their wrong interpretations. Bars should be ideal and correspond with your data points.
6. Observe your fonts and elements
Fonts emphasize a point, so you need to be careful how you use them. Only use them when it's necessary to nail a point. Otherwise, leave the fonts alone to breathe. This is one Data Visualization design principle no one will tell you about.
7. Pay attention to your colors
Want to know the best data visualization color best practices? Do not conflict colors in your data points under the guise of visualization. Use a single color to typify a specific type of data and be consistent. Avoid creating patterns with colors and don't use more than six colors in a single layout.
8. Know how you label
Proper labeling makes for an impressive visualization. But while at it, ensure your labels are visible and appropriately done. Also, label lines directly and corresponding to your data points. Be careful not to overlabel as it puts off the whole design.
9. Keep a note on how you order
Like labeling, ordering helps make sense of visualization. It's one of the Data Visualization guidelines. If you must order, make it logical and evenly. Make sure the ordering of your items conforms with that of your chart.
10. Highlight key insights
Why let the audience struggle with key insights when you can highlight them. Insights are the takeaways of a party. Therefore, you need to make sure your audience gets the souvenir before leaving.
Conclusion
Data is more appealing when visualized. The human brain internalizes visual data more than textual data. But to make a stronger case for your visual data presentation, you should do more than just present the data but also imbibe the several Data Visualization and BI best practices.
Do you think you have what it takes to kick your Data Visualization game up a notch? Begin your Visualization journey by enrolling in our Tableau Certified Associate Course right now. When done with that, enhance your data storytelling skills with our Tableau Certified Professional Course.
Do you love this post? Share with your friends on social media right away. Quickly subscribe to our newsletter for more Data Visualization tips, tricks, and best practices.